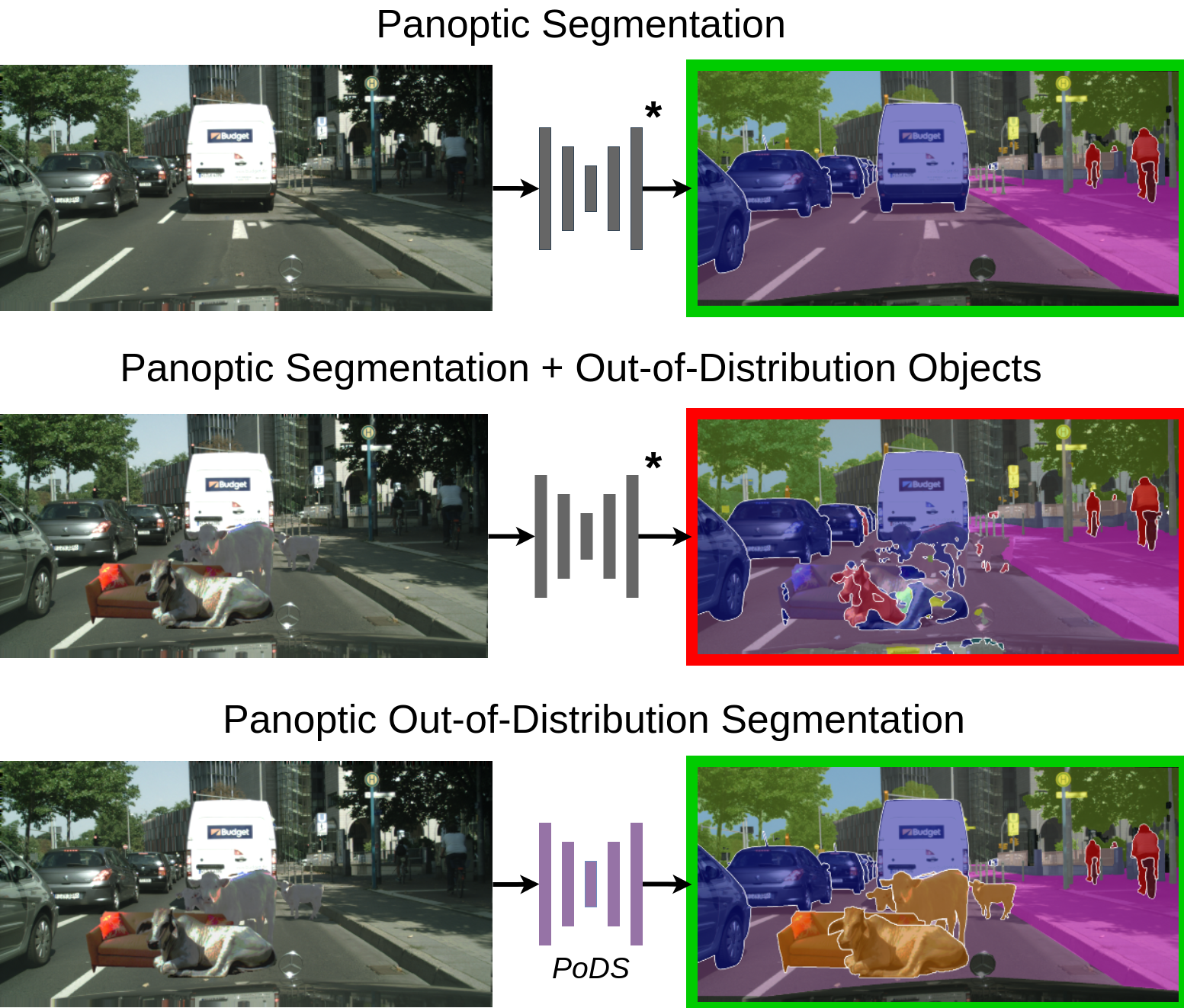
Deep learning has led to remarkable strides in scene understanding with panoptic segmentation emerging as a key holistic scene interpretation task. However, the performance of panoptic segmentation is severely impacted in the presence of out-of-distribution (OOD) objects i.e. categories of objects that deviate from the training distribution. To overcome this limitation, we propose Panoptic Out-of Distribution Segmentation for joint pixel-level semantic in-distribution and out-of-distribution classification with instance prediction. We extend two established panoptic segmentation benchmarks, Cityscapes and BDD100K, with out-of-distribution instance segmentation annotations, propose suitable evaluation metrics, and present multiple strong baselines. Importantly, we propose the novel PoDS architecture with a shared backbone, an OOD contextual module for learning global and local OOD object cues, and dual symmetrical decoders with task-specific heads that employ our alignment-mismatch strategy for better OOD generalization. Combined with our data augmentation strategy, this approach facilitates progressive learning of out-of-distribution objects while maintaining in-distribution performance. We perform extensive evaluations that demonstrate that our proposed PoDS network effectively addresses the main challenges and substantially outperforms the baselines.